LEGO Advances Automated Physical Design
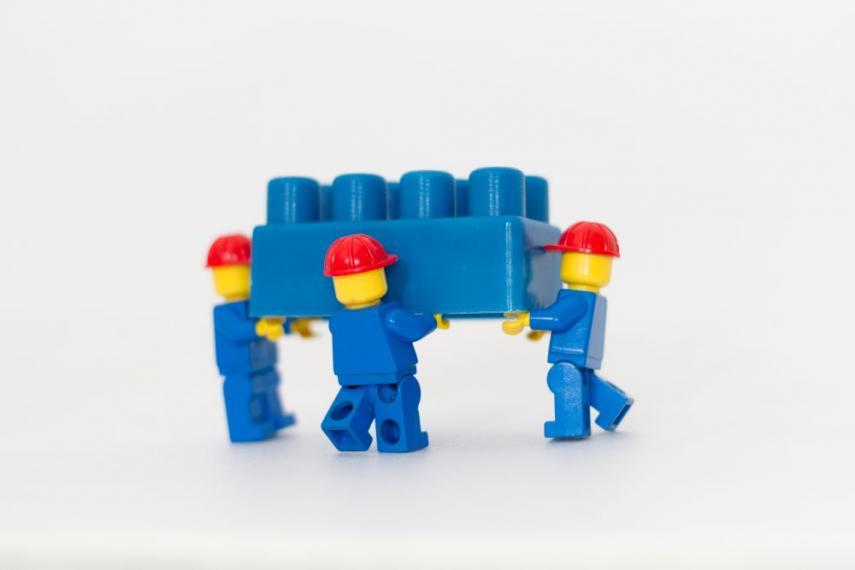
More than a childhood toy, LEGO leads to breakthrough in automated design of physical objects.
LEGO could provide the key to advancing automated architecture, landscape design, and manufacturing. Those oh-so-familiar colourful, interlocking, plastic bricks from our childhood are driving the automation of physical designs. In machine learning, generative models are used to train machines to become familiar with the natural features of objects within a dataset. Systems can then automatically create a new desired form through a series of sequential steps. Generative models are often used in the automatic creation of digital items, such as images or text, but so far, their application for physical objects is limited.
Generative Models in Machine Learning
University of Guelph engineering undergraduate student Rylee Thompson is exploring machine learning generative models for automated physical designs in conjunction with U of G-Vector Institute associate professor Graham Taylor, PhD student Terrance DeVries and postdoc Elahe Ghalebi. The researchers used LEGO as an analogue for the design of modular structures with sequential assembly. LEGO is complex enough to simulate real-world design and there is a large amount of user-created structures that can be used as a source of training data.
Building a LEGO Structure
The researchers sought to train machines to design human-like LEGO structures. They began by representing LEGO structures graphically through a series of nodes (points representing the LEGO bricks) and edges (lines representing the connections between the bricks). They then developed a machine learning model to make decisions regarding the type and direction of edges, reflecting the sequence of actions a human might follow when building a LEGO structure. To train the machine, the researchers used a dataset where 12 human subjects had created 360 different structures out of LEGO. They then mathematically tested the effectiveness of their model and found that machines were effective at automatically producing visually compelling LEGO designs.
“It’s impressive that an undergraduate student led a paper like this, which has important implications for machine learning,” says Taylor. “We can expand on this work by sourcing a larger-scale dataset that includes a wider variety of brick types and LEGO structures. Doing so will help us assess the stability of the computer-generated structures, which is crucial to ensure these structures are safe.”
G. Taylor is an associate professor in the School of Engineering and holds a Tier 2 Canada Research Chair in Machine Learning.
This work was supported by the Natural Sciences and Engineering Research Council (NSERC), the Canada Foundation for Innovation (CFI), Compute Canada, and the Vector Institute for Artificial Intelligence.
Thompson R, Ghalebi E, DeVries T, Taylor GW. Building LEGO using deep generative models of graphs. NeurIPS Workshop on Machine Learning for Engineering Modeling, Simulation, and Design. 2020 Dec 11.